Search & Algorithmic Bias: How Search Engines Shape Our Reality
- Eugenie Shek
- Feb 2
- 8 min read

Search engines like Google have become the primary gateway to information, influencing what we read, learn, and believe. With billions of searches conducted daily, these algorithms play a critical role in shaping our understanding of the world. While many assume search results are neutral and objective, research shows that search engines can reinforce societal biases, prioritize commercial interests, and even contribute to misinformation.
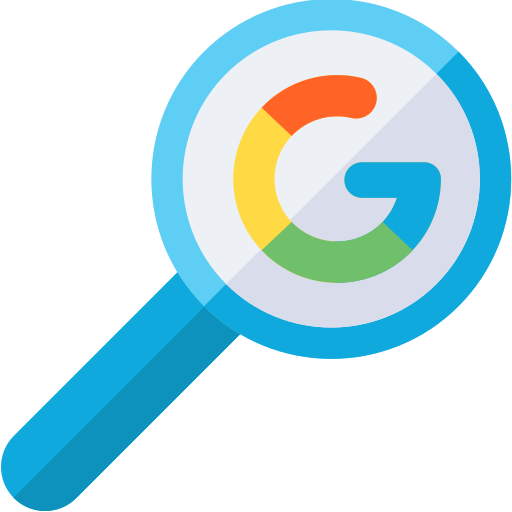
This article explores the inner workings of Google Search, shedding light on how search rankings are determined, the challenges of filtering misinformation, and the unintended consequences of algorithmic bias. By analyzing Google’s search algorithms and insights from Algorithms of Oppression by Dr. Safiya Umoja Noble, we will examine how search engines impact knowledge, culture, and social equity. Understanding these influences fosters a more ethical and inclusive digital landscape.
How Google Search Works: A Behind-the-Scenes Look
Google Search is the gateway to the world’s information, answering trillions of queries every year. The video “Trillions of Questions, No Easy Answers” provides a fascinating behind-the-scenes look at how Google processes search queries, ranks results, and constantly improves its search algorithms.
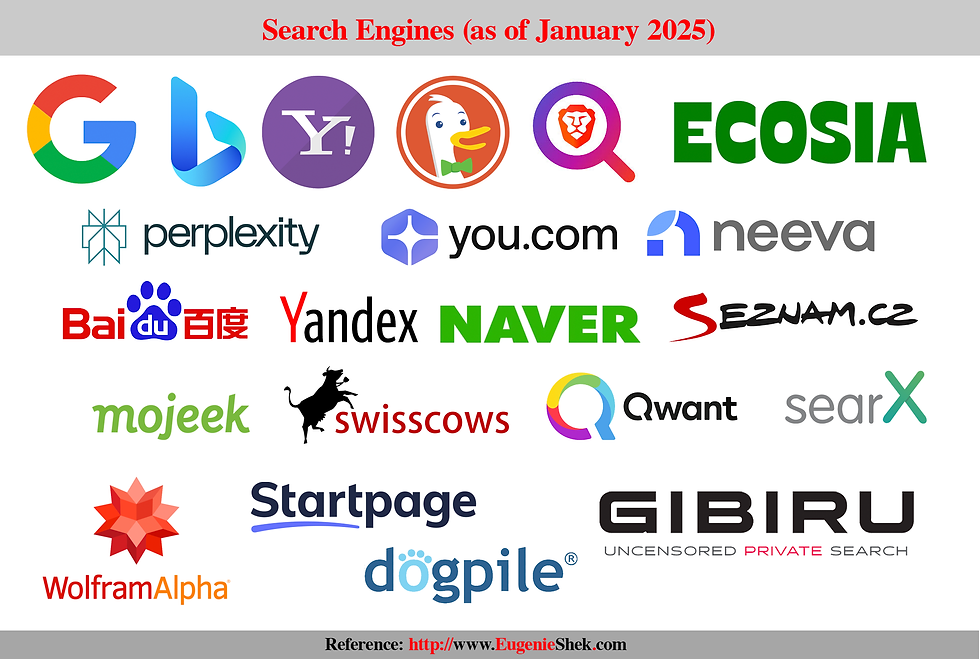
However, there are many search engines out there except Google. Statistically, as of January 2025, Google dominates the global search engine market, holding approximately 89.62% of the market share across all devices. Its closest competitor, Bing, accounts for about 4.04% of the market. Other search engines like Yandex and Yahoo! hold smaller shares, at 2.62% and 1.34% respectively.
General Search Engines (Google Alternatives)
1. Bing – Microsoft’s search engine with AI-powered features.
2. Yahoo! Search – Uses Bing’s search technology.
3. DuckDuckGo – Focuses on privacy and doesn’t track users.
4. Brave Search – Privacy-focused, independent search index.
5. Ecosia – Environmentally friendly, plants trees with ad revenue.
AI-Powered & Next-Gen Search Engines
6. Perplexity AI – AI-driven search with conversational responses.
7. You.com – Customizable, AI-enhanced search engine.
8. Neeva (Discontinued) – Ad-free, AI-powered search.
Regional Search Engines
9. Baidu – The dominant search engine in China.
10. Yandex – Russia’s largest search engine.
11. Naver – Popular in South Korea.
12. Seznam – Czech Republic’s leading search engine.
Privacy-Focused & Decentralized Search Engines
13. Mojeek – Fully independent search engine with its own index.
14. Swisscows – Privacy-focused, family-friendly search engine.
15. Qwant – Privacy-centric search engine from France.
16. Searx – Open-source metasearch engine aggregating results from multiple sources. (discontinued but available under GNU v3)
Specialized & Alternative Search Engines
17. Wolfram Alpha – Computational knowledge engine.
18. Startpage – Provides Google results but removes tracking.
19. Gibiru – Uncensored and anonymous search engine.
20. Dogpile – Metasearch engine aggregating results from Google, Yahoo, and Bing.

Understanding Google Search
Google’s mission is to organize the world’s information and make it universally accessible and helpful. Every search begins with a user query, but the challenge lies in interpreting intent and delivering the most relevant results. To ensure accuracy, Google’s algorithms analyze factors like keyword relevance, page quality, and user experience. However, Google Search and OpenAI serve different purposes and use various approaches to handling data:
Google Search: A Vast Index of the Web
Google Search does not have a centralized database like OpenAI. Instead, it crawls and indexes billions of web pages from across the internet.
When a user searches, Google retrieves relevant pages from its vast search index rather than a pre-trained dataset.
It continuously updates its index, ranking pages based on relevance, freshness, and authority.
Google does not store all website content permanently—it dynamically fetches and ranks results in real time.
OpenAI: Pre-trained Language Models
OpenAI models, like ChatGPT, are trained on large datasets, including books, articles, and licensed/public data.
Unlike Google, OpenAI does not browse the web in real time. Instead, it generates responses based on patterns learned during training.
OpenAI’s models are static until updated, meaning they do not have live access to new or updated web content.
Key Differences between Google Search and OpenAi:
Feature | Google Search | OpenAI (e.g., ChatGPT) |
Data Source | Indexed web pages | Pre-trained dataset |
Real-Time Updates | Yes (web crawling) | No (static model until retrained) |
Storage | Web index (not a database) | Compressed knowledge from training data |
Access to New Info | Instantly | Only when retrained |
While Google has a massive index of the internet, it does not store or process data in the same way as OpenAI’s AI models. If you need real-time information, Google Search is better. AI models like OpenAI’s are more valuable if you need summarised knowledge and contextual reasoning.
Challenges in Search Ranking

With the ever-changing nature of the internet, Google continuously updates its ranking systems to filter out misinformation and provide trustworthy content. The video highlights the rigorous testing and improvements made to search algorithms to enhance the user experience. Google’s ranking process considers factors such as page authority, content freshness, and mobile-friendliness to ensure users find what they need.
Combatting Misinformation
Google employs strict policies and AI-driven tools to fight misinformation and rank authoritative sources higher. The video discusses how Google refines search results to reduce the spread of fake news while ensuring diverse perspectives remain accessible.
A Google documentary | Trillions of questions, no easy answers (58:10)
The video provides an insightful glimpse into the complexities of Google Search, revealing the technology, human effort, and innovation behind delivering relevant and accurate search results.
About the book: Algorithms of Oppression: How Search Engines Reinforce Racism
In the book Algorithms of Oppression: How Search Engines Reinforce Racism, Dr. Safiya Umoja Noble critically examines how search engines, particularly Google, perpetuate systemic biases and reinforce racial stereotypes. She argues that algorithms are not neutral but reflect societal prejudices, often amplifying discrimination against marginalized communities. This eye-opening book highlights the dangers of relying solely on automated systems for information retrieval and decision-making.
The Myth of Neutrality in Search Engines
Many people assume that search engines provide objective and unbiased results. However, Noble debunks this myth by demonstrating how algorithms prioritize commercial interests and dominant cultural narratives, often at the expense of racial minorities. She provides concrete examples where racist and sexist search results appear at the top of Google searches, illustrating how technology can perpetuate inequality.
How Search Algorithms Reinforce Racial Bias
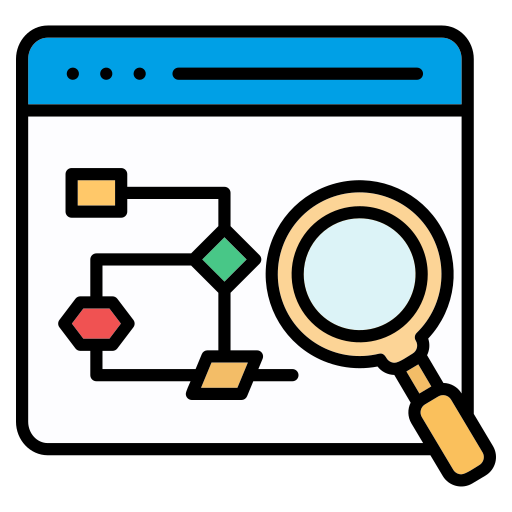
Noble’s research reveals that multiple factors, including corporate advertising, historical biases, and societal attitudes shape search algorithms. She explains how Google’s ranking system prioritizes content that receives high engagement, which often amplifies harmful stereotypes. For example, she found that when searching terms related to Black women, results often included hypersexualized or negative portrayals, reflecting long-standing racial biases embedded in digital platforms.
The Role of Corporate Interests

Search engines like Google operate on an advertising-driven model, where paid content influences visibility. Noble argues that this structure prioritizes profit over fairness, enabling misinformation and biased content to dominate search results. This model disproportionately affects marginalized communities, whose histories and realities are often misrepresented or ignored. She critiques the lack of accountability from tech companies and calls for greater ethical oversight.
The Impact on Society and Decision-Making
Noble’s analysis extends beyond search engines, exploring how algorithmic bias influences hiring practices, law enforcement, and education. She warns that as AI-driven decision-making becomes more prevalent, unchecked biases can have real-world consequences, reinforcing discrimination in areas like employment, policing, and healthcare. The book urges readers to question the role of technology in shaping societal norms and policies.
Solutions and Call to Action

Noble advocates for increased regulation of tech companies, ethical AI development, and greater diversity in the tech industry. She encourages policymakers, educators, and activists to challenge algorithmic discrimination and push for more transparent and equitable digital systems. Society can work towards a more just and inclusive technological future by promoting digital literacy and awareness.
Algorithms of Oppression is a must-read for anyone interested in the intersection of technology, race, and social justice. Noble’s research underscores the urgent need to address algorithmic bias and rethink the role of search engines in shaping knowledge. As technology continues to evolve, ensuring fairness and inclusivity in digital spaces remains a critical challenge.
How many companies are developing LLM? (Large Language Models)
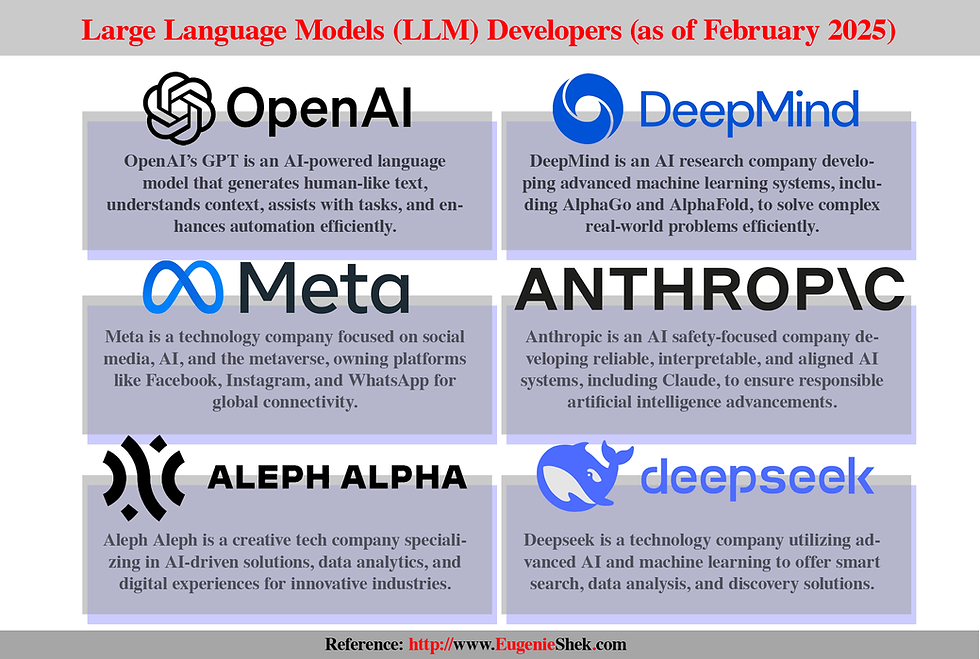
As of February 2025, numerous companies are developing large language models (LLMs) similar to OpenAI’s GPT series. These models are designed to understand and generate human-like text, with applications across various industries. Notable organizations in this field include:
Open AI: OpenAI is an artificial intelligence research organization focused on developing safe and advanced AI technologies. Founded in December 2015 by tech leaders like Elon Musk, Sam Altman, Greg Brockman, Ilya Sutskever, John Schulman, and Wojciech Zaremba, OpenAI aims to ensure that artificial general intelligence (AGI) benefits all of humanity. Language Models: OpenAI is best known for its GPT (Generative Pretrained Transformer) models, such as GPT-3 and GPT-4, which are advanced LLMs capable of tasks like text generation, translation, summarization, and even engaging in human-like conversation. [Notable Models] GPT-3, GPT-4, GPT-5 (anticipated) [Key Focus] General-purpose language models capable of tasks like conversation, text generation, and problem-solving
Google DeepMind: Developed the Gemini family of multimodal LLMs, serving as successors to their previous models, LaMDA and PaLM 2. Gemini powers Google’s AI chatbot and directly competes with OpenAI’s GPT-4. [Notable Models] Bard, Gemini (previously known as Bard) [Key Focus] Pushing the boundaries of deep learning for natural language understanding and generation, as well as AI safety.
Meta (formerly Facebook): Created the LLaMA series of LLMs, with LLaMA 3 being the latest iteration. These models are open-source and have been utilized in various research and commercial applications. [Notable Models] LLaMA (Large Language Model Meta AI)
[Key Focus] Open-source language models aimed at research and democratizing access to powerful AI tools.
Anthropic: Founded by former OpenAI employees, Anthropic has developed the Claude series of LLMs, focusing on safety and alignment in AI systems. Claude has been recognized for its advanced reasoning capabilities. [Notable Models] Claude (Claude 1, 2, 3, and expected future versions) [Key Focus] AI safety and alignment, focusing on developing AI models that are interpretable, ethical, and trustworthy.
Aleph Alpha: A European AI startup that developed the Luminous model, known for its multimodal capabilities, allowing it to process and generate both text and images. Luminous has been applied in various enterprise solutions. [Notable Models] Luminous [Key Focus] Aiming to create advanced language models that work in multiple languages and industries, with an emphasis on interpretability.
DeepSeek: A Chinese AI company that recently introduced DeepSeek R1, a chatbot model comparable to leading U.S. models but developed at a significantly lower cost. This release has been described as a “Sputnik moment” for AI, highlighting the rapid advancements in the field.
Mistral AI
• Notable Models: Mistral 7B, Mistral Mix, and other models
• Key Focus: Developing open-weight language models, focusing on performance and efficient scaling.
Cohere
• Notable Models: Command R
• Key Focus: Specializing in efficient, scalable language models tailored for applications in business and industry.
X (formerly Twitter)
• Notable Models: Various proprietary models related to content moderation and user interaction.
• Key Focus: Incorporating language understanding for social media, conversational AI, and user-centric recommendations.
Huawei
• Notable Models: PanGu-α (PanGu AI)
• Key Focus: China-based tech giant creating large language models for applications in AI, cloud, and computing services.
Baidu
• Notable Models: Ernie (Enhanced Representation through Knowledge Integration)
• Key Focus: China-based company specializing in AI and LLMs, focusing on multilingual understanding and search technologies.
Samsung Research
• Notable Models: Bixby AI and various language models for consumer devices
• Key Focus: Developing conversational agents for Samsung products, with a growing focus on LLMs for customer interaction.
EleutherAI
• Notable Models: GPT-Neo, GPT-J, GPT-NeoX
• Key Focus: Open-source LLMs aiming to provide the research community with highly scalable models.
Stability AI
• Notable Models: StableLM
• Key Focus: Building open-source language models, similar to their focus in generative art (Stable Diffusion).
In addition to these organizations, several other companies and research institutions are actively developing LLMs, contributing to the rapid evolution of AI language models. The landscape is dynamic, with new developments emerging regularly.
Comments